Addressing the complexity of AI and edge operationalization
Article by Bertrand d’Herouville, Senior Specialist Solutions Architect, Edge at Red Hat
With today’s artificial intelligence (AI) hype, there can be a lot to digest. Each day brings a new product, new model or a new promise of fault detection, content generation or other AI-powered solution. When it comes to operationalizing an AI/machine learning (ML) model, however, bringing it from training to deployment at the edge is not a trivial task. It involves various roles, processes and tools, as well as a well-structured operations organization and a robust IT platform to host it on.
AI models are now flourishing everywhere but this raises the question: how many of them are operationalized solutions with a robust AI model training, versioning and deployment backend?
How long does operationalization take?
This question is not new. According to a recent Gartner survey, it could take organizations anywhere between 7 – 12 months to operationalize AI/ML from concept to deployment. Technical debt, maintenance costs and system-level risks are just a few things that must be managed throughout the operationalizing process.
Key challenges in AI/ML operationalization
Operationalizing an AI/ML model from a proof-of-concept phase to an industrial solution is full of challenges. This operationalization is very similar to the creation of a high-quality industrial product. Specifically, a chain of trust needs to be created to create a reliable and high quality solution.
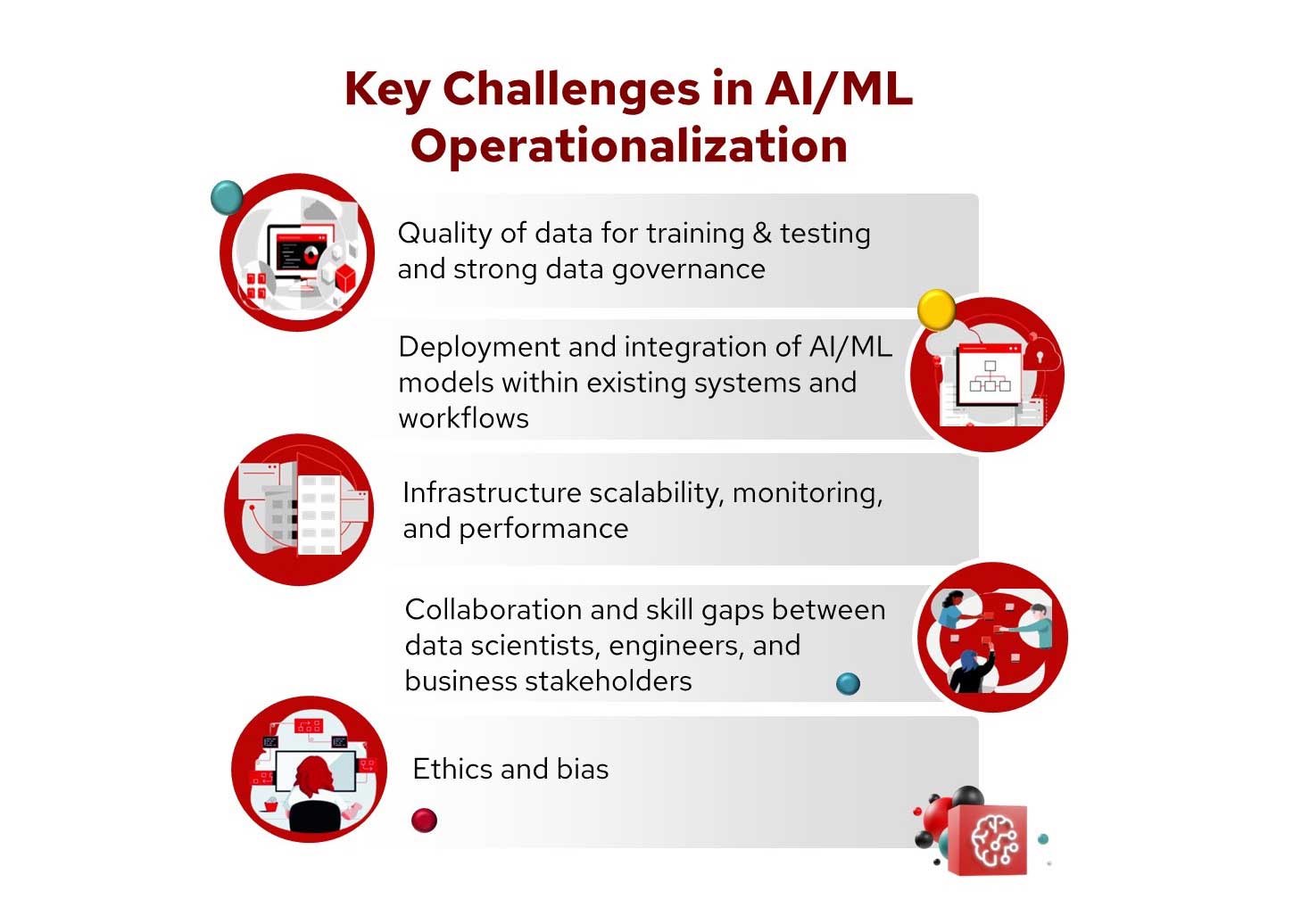
Data quality
Creating a robust AI model includes having clean and relevant data for training and testing. At its core, the underlying requirement is having a strong data governance, which includes data privacy, security and compliance with all relevant regulations. This can add an enormous amount of complexity and increased infrastructure needs.
Model deployment and integration
Integrating AI/ML models within existing systems and workflows can be technically challenging, as most modern software relies on a continuous integration and continuous deployment (CI/CD) approach. Due to this, it is important to implement pipelines for AI models that include extensive automation and monitoring facilities.
Infrastructure scalability, monitoring and performance
Making sure that AI/ML models are trained in scaling to handle large volumes of data and high-velocity data streams is critical. For that, an architecture which both data scientists and IT operations can monitor and maintain will mean that the platform can be fixed or upgraded when needed. This includes tooling for building and maintaining the necessary infrastructure—including computational resources, data storage and ML platforms—and selecting and integrating the right tools for data processing, model development, deployment and monitoring.
Collaboration and skill gaps
Bridging the gap between data scientists, engineers and business stakeholders is key to fostering alignment and effective collaboration.
The widespread use of specialized tools for different functions can impede this process. Each group often relies on unique tools and terminology, which can create barriers to integration, especially in machine learning operations (MLOps). In an ideal world, models will move smoothly through the process from training through to deployment out to edge devices. It may even be tempting to utilize a single system or platform to achieve this ideal process. To foster more effective collaboration, however, it may be a better approach to create platforms and tools that integrate well with each other, so each team can use their preferred systems over a single solution that may not fit their specific needs.
Bridging skill gaps is also crucial in this process. Organizations will often have a division of expertise where data scientists, engineers and business stakeholders possess distinct skills and knowledge. This division can cause problems when models are transferred between teams, as the necessary skills and understanding may not be uniformly distributed. Addressing these gaps requires ongoing education and cross-training, so all team members have a basic understanding of each other’s roles and tools. By investing in cross-team skill building and training, model development and deployment processes will be smoother and more effective.
Ethics and bias
Making sure that AI/ML models are fair and do not perpetuate existing biases present in the data involves implementing ethical guidelines and frameworks to govern the use of AI/ML. Then the ethics and bias guidelines must be implemented as metrics in order to create a reliable infrastructure for evaluation and monitoring. The quality of a model has to be evaluated against these metrics and the results be compared to pre-defined references.
Roles involved in the AI/ML lifecycle
Operationalizing AI/ML involves various roles, each contributing critical skills and knowledge. These roles differ from regular IT DevOps ones as they are demanding consumers of IT infrastructures, using specific tools for the AI modeling and facing the same challenges in delivering the AI models like delivering a new software version.
One of the more well-known ones is the data scientist. This term however, is too generic, and the tasks for AI/ML deployment splits to different individuals with specific skills. For the data collection, cleaning, and preparation for model training, it would require a data scientist.
An MLOps engineer would specialize in the deployment and maintenance of AI models. This role involves specific skills and tools that are not necessarily the same as those used in AI model training. This is generally where a company struggles to operationalize their AI model, when very skilled data scientists are asked to deploy AI models. Deployment specifically is a skill that falls under that of a MLOps engineer, as well as monitoring, scaling models into production and maintaining performance.
Creating an AI/ML model involves an AI engineer, which will train and deploy models running the latest algorithms created by AI scientists. Once the model is created it is pushed into a storage system to be available for containerized application.
App developers integrate AI/ML models into applications, ensuring seamless interaction with other components.
IT operations manage the infrastructure, ensuring necessary computational resources are available and efficiently utilized.
Business leadership sets goals, aligns AI/ML initiatives with business objectives and ensures necessary resources and support exist to continue operationalizing AI/ML solutions.
As you can imagine, coordinating these roles effectively is crucial for managing the complexities and technical debt associated with AI/ML operations. This coordination cannot be done just by defining procedures and abstract workflows. This needs to rely on a common set of tools with specific user interfaces for each role to give a shared but readable view on the AI operations.
How Red Hat can help
Red Hat OpenShift AI provides a flexible, scalable AI/ML platform that helps you create and deliver AI-enabled applications at scale across hybrid cloud environments.
Built using open source technologies, OpenShift AI provides trusted, operationally consistent capabilities for teams to experiment, serve models and deliver innovative apps.
We’ve combined the proven capabilities of OpenShift AI with Red Hat OpenShift to create a single AI application platform that can help bring your teams together and help them collaborate more effectively. Data scientists, engineers and app developers can work together on a single platform that promotes consistency, improves security, and simplifies scalability.
Red Hat Device Edge is a flexible platform that consistently supports different workloads across small, resource-constrained devices at the farthest edge and provides operational consistency across workloads and devices, no matter where they are deployed.
Red Hat Device Edge combines:
- Red Hat Enterprise Linux
Generate edge-optimized OS images to handle a variety of use cases and workloads with Red Hat’s secure and stable operating system.
- MicroShift
Red Hat’s build of MicroShift is a lightweight Kubernetes container orchestration solution built from the edge capabilities of Red Hat OpenShift.
- Red Hat Ansible Automation Platform
Red Hat Ansible Automation Platform helps you quickly scale device and application lifecycle management.