Generating AI May Be Easier Than You Think
AI combined with serverless cloud computing is the way forward.
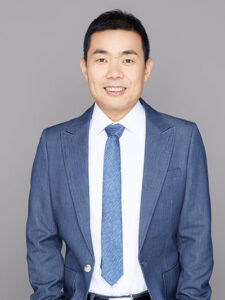
Since ChatGPT first caught the public’s attention late last year, almost every organisation has been getting into or creating some form of generative AI. More recently, we have seen conversations maturing to discussions on how exactly we can make AI work, especially given the high cost and complexity it requires to live up to the expectations that the hype has generated.
Let’s take a step back first to examine how we arrive at AI. AI is promising because it can be wise. It spots things we may miss or even disregard because it has more capacity and consistency. But fundamentally, data is the foundation for AI.
That means we need to ensure data is properly processed and protected. It is the lifeblood of not just the entire IT infrastructure, but also the basis of all innovation that comes from real humans or artificial intelligence. As part of the underlying infrastructure that powers generative AI, databases have evolved to cater to the demands of corporations in the generative AI era. How effective your AI is boils down to how you are managing data using the right database.
Common Database Models
There’s a type of database called Online Transaction Processing (OLTP), which supports online transaction processing. This basically enables businesses to transact concurrently – for example in online banking, shopping, and so on – and as data accumulates in the database, you can derive value out of the data pool.
We also have On-Line Analytical Processing (OLAP) – which enables organisations to do fast, interactive, and powerful analytics from the data because it helps consolidate data from multiple sources beyond just the transactional ones.
For example, a retailer can combine data in its inventory and what it has in stock with another dataset of what customers are buying to provide intelligence on the need to increase production of a particular item over another due to higher demand.
Another database model family called NoSQL is popular because it helps sort unstructured data unlike the two above.
An emerging database model for AI
But in the emerging age of AI, we expect the vector database model to be the most transformative.
A vector database is used to handle ‘‘intelligent workloads’’ with large language models for embedding and storing those millions of high-dimensional vectors – think of unstructured data such as documents, images, audio recordings, videos and so on which is on track to account for over 80 percent of all data worldwide by 2050 – to enable the semantics expected of AI, that is understanding the underlying context and nuances rather than just the meaning.
AI is ultimately about making sense of the data, and you can’t make sense without using a vector database. It is a key requirement to increase the industry-specific knowledge of large language models, which is one of the largest constraints facing generative AI models.
Alibaba Cloud has enhanced its full range of database solutions – including cloud-native database PolarDB, cloud-native data warehouse AnalyticDB, and cloud-native multi-model database Lindorm – with its proprietary vector engine. Enterprises can now input sector-specific knowledge into their vector databases, enabling them to build and launch generative AI applications.
Let’s use an actual business case to illustrate the power of a vector database.
One of Alibaba Cloud’s customers – a major online gaming company from Southeast Asia – is using Alibaba Cloud’s database solutions to create intelligent Non-Player Characters (NPCs) that can engage a lot more authentically with human players because they are not “reading” a scripted set of lines, but reacting based on real-time understanding of what players are communicating.
Dollars and sense
The promise of AI is not just limited to gaming or even making sense of unstructured data.
AI can manage the database itself. For example, when storage is running low, AI can alert system administrators to watch out for storage requirements and ask if there is a need to extend storage space. AI can automatically scale the storage space if permission is given. The same functionality can be applied to CPU capacity, memory capacity, and other functions.
This capability is handy given the move towards serverless cloud computing. As the name suggests, serverless means that there is no longer a need to worry about servers behind the service you’re using.
In the past when one purchased a cloud service product, a provision had to be made for a set of servers. For example, four cores and eight gigabytes of memory came with a cost. When one provisions a server that has more capacity than the actual workloads require, it ends up wasting server resources.
Serverless computing is designed to address this challenge and ensure that the server capacity used by the cloud service precisely matches the needs of the workloads. It adapts to the dynamic change in the workloads. However, if workloads are changing dynamically over time, then the serverless way might end up costing you more.
The best of both worlds
By combining AI with serverless cloud computing, we are getting the best of both worlds. That’s why Alibaba Cloud has also made its key AI-driven database products serverless. Customers pay only for the number of resources required and AI is there to guide and augment decision-making capabilities in dealing with sudden peaks in demand or a very dynamic workload.
How you make AI work for you by working with the right databases will determine whether your organisation rides this AI trend towards success or is left behind.